Curious about what keeps experts, CEOs and other decision-makers in the Intelligent Document Processing (IDP) space on their toes? Get food for thought on IDP-related topics from the industry’s leading minds.
In this opinion piece, Marie-Pierre Garnier, VP of Marketing & Communications at Intelligent Document Processing (IDP) vendor Cortical.io, explains why, according to analyst firm Gartner, only 48% of AI proofs of concept (POC) make it into production, and what companies should do to maximize the chances of success for their AI projects.
Why the fear of missing out should not motivate your investment in generative AI
The hype surrounding generative AI has triggered many initiatives across organizations of all sizes, with both a non-neglectable pressure from the C-levels and an explosion of faith in the genAI’s problem-solving capabilities. According to a Gartner survey from March 2024, more than 40% of CIOs have already launched or plan to implement generative AI projects by the end of 2024. However, developing in-house AI applications and leveraging large language models (LLMs) prove to be much more challenging than most companies anticipated, with an estimated 50% of generative AI deployments in the enterprise getting stalled. This article explains how enterprises can maximize the return on investment of AI projects.
According to analyst firm Gartner, only 48% of AI proofs of concept (POC) make it into production. Why is every second AI project abandoned before completion?
- Lack of trustworthiness (biases & hallucinations): Inaccurate, incomplete data sets lead to incorrect answers, and therefore wrong decisions, sometimes even discrimination. OpenAI estimates that LLMs can be subject to hallucinations or factual errors in 30% or more of their output. The trickiness with LLMs is that they deliver wrong content without hinting at its potential inaccuracy, which makes it difficult for end-users to discern true facts from fictions.
- Concerns about security and data privacy: Deploying LLMs in the enterprise bring risks in terms of data leakage and compliancy. It is difficult to govern privacy and data protection policies in externally hosted environments, as it is difficult to assess the GDPR- and CCPA-compliance of third-party models, which are conceived as black boxes.
- Lack of AI experts: Developing an in-house AI solution requires to build a pool of specialists with a wide range of skills, including machine learning, data science and advanced analytics. The market for this kind of talents is largely dominated by demand, making it both difficult and expensive for companies to build an AI team. Additionally, generative AI applications also require new competencies from end users who often struggle crafting an adequate prompt to generate relevant responses.
- Challenging integration with legacy systems: In some organizations, legacy systems that have evolved over time make it difficult to integrate modern AI technologies. The existence of data silos constitutes a major impediment to the successful deployment of generative AI.
- Difficulty in calculating a business case with a clear ROI: Many generative AI projects are launched out of enthusiasm, without a concrete business case. The long-term resources these projects will tie up, as well as their costs, are usually underestimated.
This does not mean you should keep your fingers from AI initiatives. If well planned, AI has the potential to improve the efficiency and quality of many different work processes. So, what should you undertake to maximize the chances of success of your AI projects?
- Ensure data quality: The output of AI systems is only as good as the input. The success of an AI project depends primarily on how clean and representative the data is that flows into model training. Moving away from data silos and ensuring data consolidation into a unified system is another pre-requisite for successful generative AI projects.
- Focus on AI governance: Compliance with the AI Act, GDPR and CCPA must be guaranteed. It is just as important to establish internal AI governance to define processes, control mechanisms and ethics. Best practice is to create a Center of Excellence for AI including cross-organizational stakeholders.
- Involve business and IT: The AI project must develop into a production-ready solution with added value for the organization – that’s why you need to involve business users from the beginning. They will help you build a business case with measurable added value. You must also involve your IT experts to ensure the proper integration of the solution into the existing enterprise software architecture.
- Assess the technology fit: Generative AI is not always the answer. There are many use cases where it is even a wrong fit. First, you should conduct a market survey to identify the technologies required to address the use case in its entirety – very often generative AI will have to be combined with machine learning and natural language processing to achieve the best results.
- Weigh up the pros and cons of an in-house solution: Only develop applications with your own team when a high level of in-house expertise and customization is required, or when singularity provides a strong competitive advantage. The time frame for developing, testing and implementing in-house applications is much longer than buying a solution, even if it needs to be fine-tuned – think of years versus months.
- Leverage external expertise: In many cases, instead of experimenting with raw AI algorithms, it is advisable to draw on the expertise of AI software companies that have already gone through the experimental phase and offer a ready-made, tested, scalable solution.
Conclusion
Even if the pressure from the media is urging management to start generative AI initiatives, the economic viability of each project should always be the top priority. Anyone starting with GPT or other LLMs must be prepared for a long experimental phase that ties up resources in the long term – with no guarantee of success. In many cases, instead of experimenting with raw AI algorithms, it is advisable to draw on the expertise of software vendors to shorten time to value – and more speedily ride the slope of AI enlightenment.
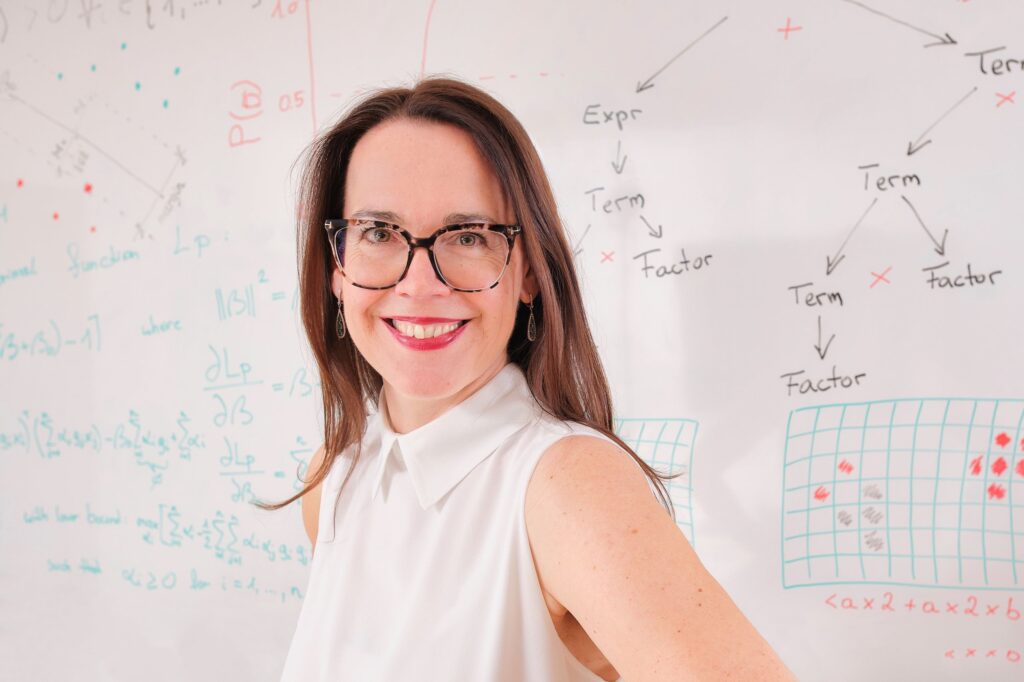
About the Author
Marie-Pierre Garnier is the VP of Marketing & Communications at Cortical.io and has been following the artificial intelligence and natural language processing markets for more than 10 years. She is particularly interested in the sustainability aspects of business software solutions and has written several articles about the subject. In her previous role at the Information Retrieval Facility, MP was facilitating the knowledge transfer between industry search experts and information retrieval scientists.
📨Get IDP industry news, distilled into 5 minutes or less, once a week. Delivered straight to your inbox ↓