Curious about what keeps experts, CEOs and other decision-makers in the Intelligent Document Processing (IDP) space on their toes? Get food for thought on IDP-related topics from the industry’s leading minds.
In this opinion piece, Maxime Vermeir, Senior Director of AI Strategy at IDP vendor ABBYY, explains the current move from large language models (LLMs) to small language models (SLMs).
The pace of progress in language model capabilities hasn’t slowed down, dazzling us with new possibilities. Intelligent document processing (IDP) stands as a prime beneficiary of these leaps, with IDC estimating the worldwide IDP market will grow at a CAGR of 28.7% from $1.75 billion in 2022 to $6.17 billion in 2027. Large language models (LLMs), specifically, are not without their share of questions about the value and advances they bring, especially when weighed against speed, performance, and costs.
In fact, there was early confusion to laymen that LLMs were similar to IDP due to the mere fact that both recognize data. However, those of us knowledgeable in information technology know that IDP plays a vital role in training LLMs with high-quality data. IDP uses advanced AI to recognize, extract, and classify data from documents thereby enabling LLMs to summarize large volumes of data.
However, examining the cost of LLMs causes a pause for enterprise use. Depending on the type of model, costs to summarize 100,000 documents can range up to $30,000 for each query. Not sustainable for most budgets. Environmental factors are also being examined as organizations strive to meet ESG standards. A study by Cornell University showed that training one model can produce over 600,000 pounds of CO2, and another study from Indiana and Jackson State University found the carbon footprint of GPT-3 trained by different computing devices are the equivalent of a roundtrip flight from San Francisco to New York.
In response, there’s a noticeable pivot towards small language models (SLMs). These models are not only easier to train and faster to operate but also significantly lighter on the wallet and better for the environment. This shift prompts a reflective question: haven’t we walked this path before?
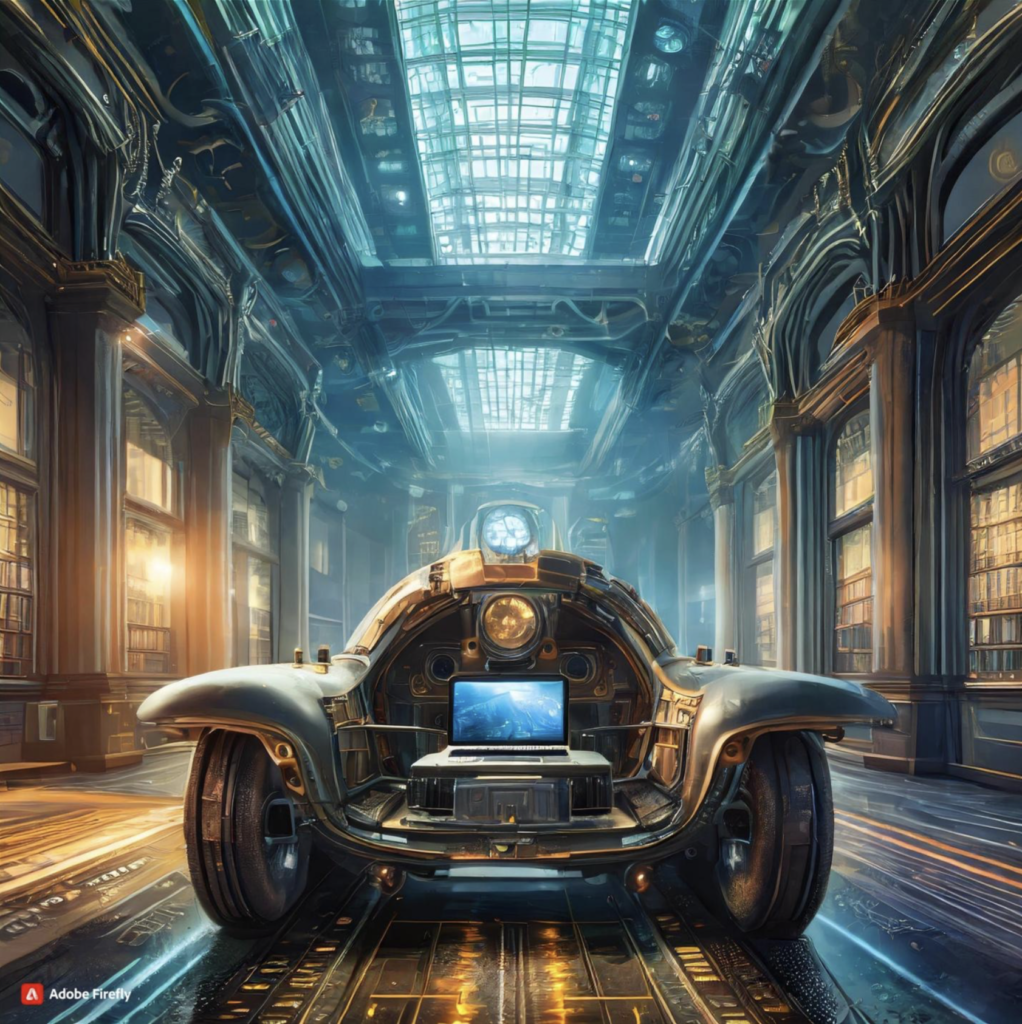
Cue my Marty McFly moment: “All right, this is an oldie, but… well, it’s an oldie where I come from.”
The last major tech shift saw machine learning aimed at tackling niche problems with highly specialized models. Each excelled in its domain.
For example, Image Semantic Segmentation has shown to be both a great technology to help self-driving cars see and identify objects on your path, and the same technology is powering your IDP platform to identify all objects within a document (tables, headings, barcodes, signatures, etc.)
Another example is clustering. Clustering algorithms group documents with similar characteristics, using features like word frequency, to organize large datasets into understandable categories without predefined labels to facilitate a faster rate of training with higher accuracy. As you might expect, it builds upon the data that came out of the previous example to do so.
The current move from LLMs to SLMs mirrors this approach. However, I see it less as a groundbreaking discovery and more as a strategic recalibration and being more purposeful with AI applications. LLMs, while transformative, represent just one of many tools in our arsenal, guiding us toward pragmatic solutions for real-world challenges.
Delving into the numbers from ABBYY’s R&D sheds light on this transition. Consider the comparison: a RoBERTa model (123M parameters) achieves, for example, 55% accuracy in an OCR task, while our SLM (1-10M parameters) hits close with 50% accuracy on our dataset. Yet, RoBERTa drags its feet in speed, delivering comparable outcomes approximately 50 times slower.
This is exactly what buyers in the market are expecting today, the improved outcomes of these advanced technologies, but without the excess and need for heavy resource consumption which is bad for both the environment and the bank.
The SLM landscape itself is evolving rapidly. The democratization of AI enables SLMs purposely built for IDP and specific document types to be deployed easily within the most commonly used intelligent automation platforms. We’ll see an increase in model variations to process more complex document formats, languages, and business processes. With innovative architectural tweaks, including the integration of transformer blocks, the dependency on voluminous data diminishes further. The perks extend beyond just swift inference; training durations have shrunk from months to mere days.
Thus, we don’t need to reverse for momentum—there’s no need for the DeLorean to hit 88 miles per hour. In IDP, the future is already here, and intriguingly, it looks a lot like revisiting the fundamentals—with a twist.
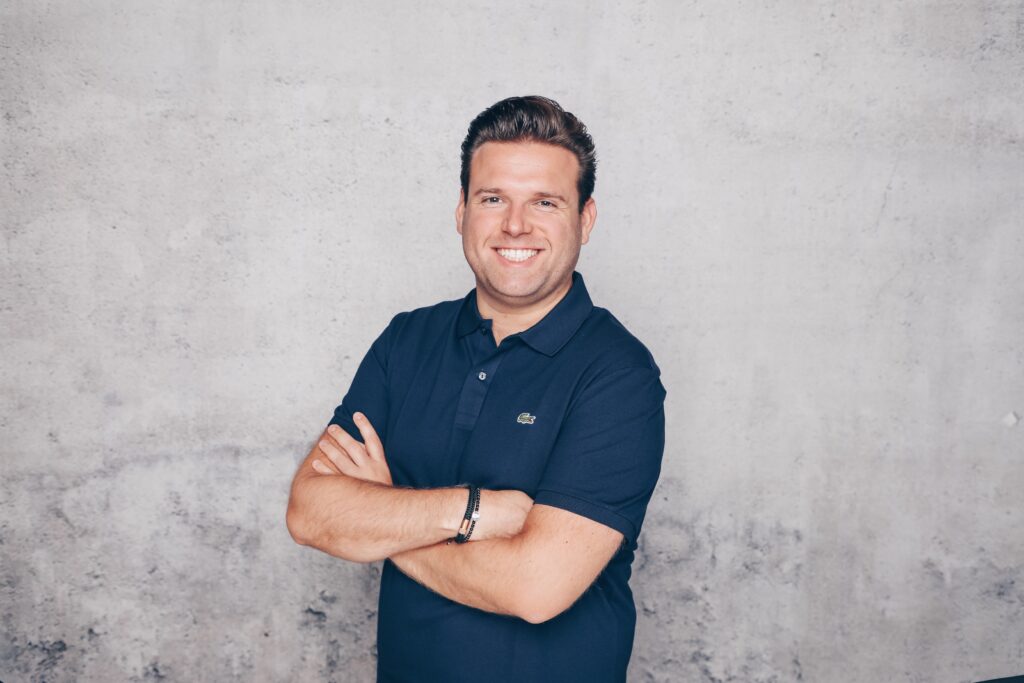
About the Author
Maxime Vermeir is Senior Director of AI Strategy at intelligent automation company ABBYY. With a decade of experience in product and tech, Maxime is passionate about driving higher customer value with emerging technologies across various industries. His expertise from the forefront of artificial intelligence enables powerful business solutions and transformation initiatives through large language models (LLMs) and other advanced applications of AI. Maxime is a trusted advisor and thought leader in his field. His mission is to help customers and partners achieve their digital transformation goals and unlock new opportunities with AI.
📨Get IDP industry news, distilled into 5 minutes or less, once a week. Delivered straight to your inbox: